Scaling AI sustainably: 4 urgent priorities
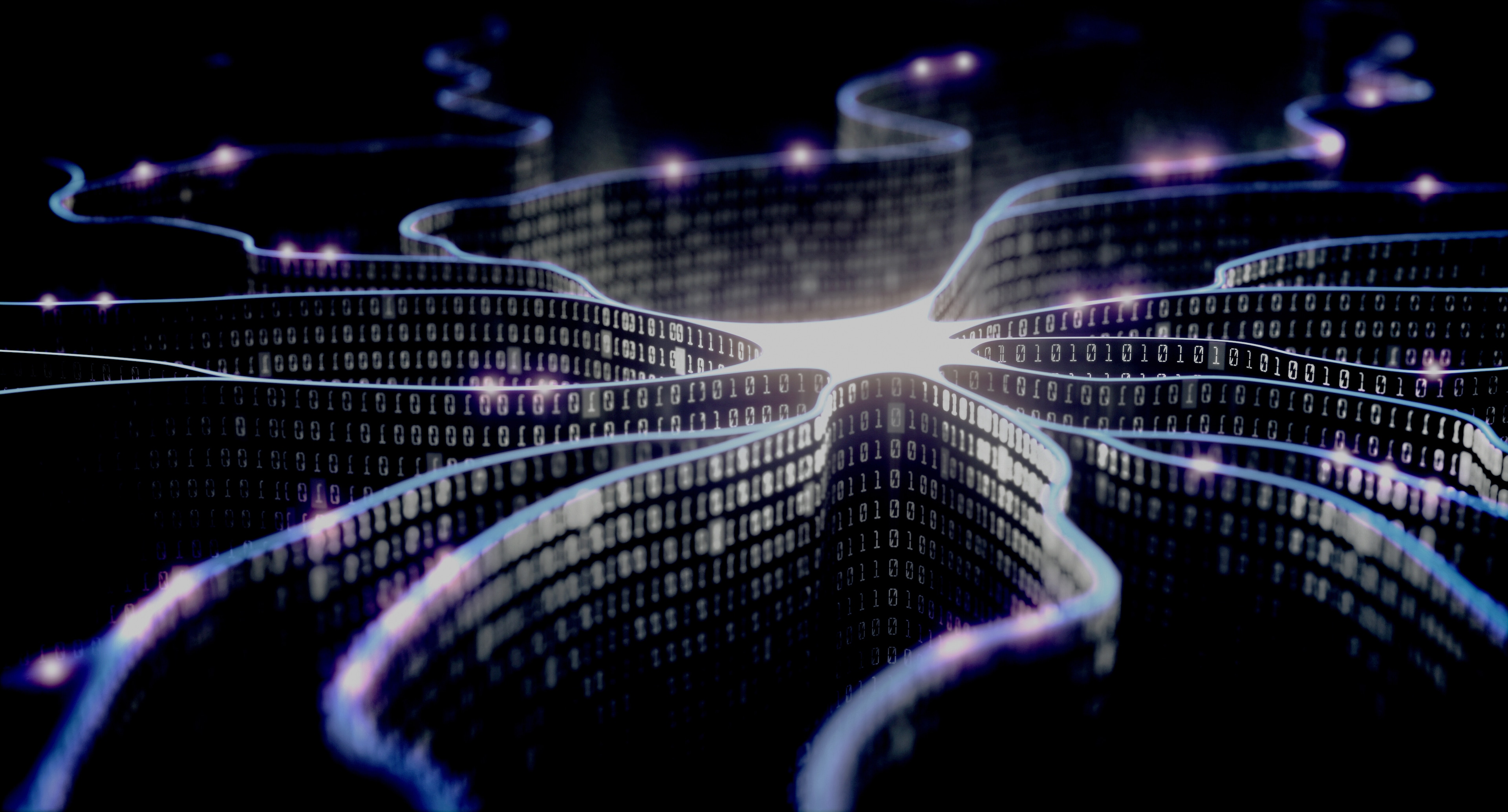
Responsible AI requires everyone to do their part
Image: Getty Images/iStockphoto
Stay up to date:
Sustainable Computing
- Organizations must evaluate whether artificial intelligence (AI) is the most efficient tool for the task, ensuring it is ethical, privacy-enabled and environmentally conscious to minimize risks and energy consumption.
- Improving efficiency at every stage of AI – from data selection to training and deployment – can dramatically reduce environmental impact.
- Governments, industries, and innovators must partner to promote sustainable energy solutions, equitable access to clean energy, and harmonized regulations that prioritize innovation and the environment.
Artificial intelligence (AI) is the opportunity of a lifetime to leverage technology for good. Yet, it presents a paradox.
It offers staggering capabilities that could unlock a more reliable, low-emissions energy future through technologies such as nuclear fusion and smart grids. However, the near-term energy consumption required for the computational cycles required to develop these technologies could exacerbate the very problem we are trying to solve.
Improving AI sustainability is both a moral obligation and a practical imperative that must motivate industry, policymakers, and end users to act swiftly. Improving AI efficiency is also in everyone’s interest, as consuming less energy and water reduces operating costs and the impact on the environment.
To be successful, we must work together, think holistically, and act urgently to devise a sustainable path forward. Here are four priorities we must embrace to scale AI responsibly.
1. Ensure AI is the right tool for the job
AI will revolutionize industries and scientific discoveries globally, but organizations and technology leaders need to use AI intentionally, given what it takes to run it. We must carefully evaluate AI, among other available tools, which may be better suited and optimized to deliver on business requirements with a lighter environmental footprint.
A quick comparison of a generative predictive tool (GPT) versus a standard search engine query illustrates this point: A single generative AI (GenAI) query consumes about 15 times more energy than one search engine query, but the intrigue surrounding GPTs could lead users to favor them. For this reason, we must assess whether GenAI is the right tool for the job.
AI isn’t needed for simple applications any more than one needs a race car to go to market. When embarking on a new AI project, organizations should develop an AI business plan that clearly lays out details such as expected business outcomes, data needs, AI model requirements, and energy and cooling demands.
Furthermore, if AI does not incorporate responsibility by design, it can present unique and serious ethical risks.
To realize value while mitigating these risks to your organization, AI must be designed to be privacy-enabled and secure, protective of human rights, inclusive and unbiased, minimize opportunities for misuse and abuse, and explainable to ensure accountability.
Can your use case effectively and efficiently account for these risks and mitigate them to promote trust? Diligently working through such considerations can help identify where alternatives to AI might be more appropriate.
2. Optimize across the entire AI lifecycle
Scaling AI sustainably requires us to pull all available levers to optimize performance in the interest of sustainability. Importantly, this is not a one-and-done step in an IT sustainability exercise. It must occur at every stage in the AI lifecycle, from data selection to model design, training, tuning and inferencing – through to the equipment’s end of life.
Although much attention has understandably been given to advancements in AI infrastructure and equipment efficiency—such as advanced chips or a 100% direct liquid cooling system architecture that can reduce power consumption by 90%—we must think beyond the equipment.
The AI lifecycle must be studied to improve software, data, energy, and resource efficiency. For example, focusing on data efficiency could involve critically evaluating a data set before feeding it into a model for training to ensure we are not wasting processing power crunching extraneous or irrelevant data.
Similarly, using pre-trained models when possible can eliminate the energy usage required to re-train a new model when an adequate model already exists.
Organizations must also implement the measurement and monitoring tools necessary to track detailed performance metrics. Surveys reveal that only 44% of enterprises actually monitor AI-related energy use – leaving environmental savings on the table.
Coupling granular monitoring with holistic thinking has already helped researchers identify opportunities to reduce emissions by up to 80% simply by adjusting the time that models are trained to align with windows where renewable energy is more plentiful.
3. Push toward a cleaner energy future, everywhere
The reality of the globe’s 24-seven energy demands requires technology innovators and policymakers to form productive partnerships and direct resources toward initiatives that spark innovation and help us rethink our roadmap to a low-carbon energy future.
This may require reevaluating preconceived notions about low-carbon energy sources such as nuclear power as part of a broader, reliable energy mix.
The developed world also has a responsibility to help developing economies decarbonize their energy grids. Many nations lack access to infrastructure for lower-carbon energy sources due to a lack of funding, sourcing constraints, and even political will.
It is more important than ever to build on the progress that many parts of the world have made in decarbonizing energy production so that everyone can sustainably access the benefits of AI.
4. Implement smart regulatory policies for responsible AI
Policymakers and the private sector must collaborate to develop smart, effective and practical regulatory policies. These policies should incentivize innovation in sustainable IT, monitor and account for power consumption and emissions related to AI, and harmonize across borders to facilitate the rapid adoption of best practices.
Adopting methodologies developed by international bodies, such as the Institute of Electrical and Electronics Engineers, should be encouraged. Although several AI regulations have emerged, the majority fail to account for environmental sustainability.
Furthermore, the stop-gap measure of enacting temporary moratoriums on data centres is not a realistic long-term solution. What is required is a holistic and comprehensive approach that prioritizes environmental sustainability in addition to broader considerations such as ethical use.
Robust public-private partnerships that prioritize radical innovation are imperative to realizing AI's full potential while safeguarding our natural resources. Leaders from government, industry and civil society must collaborate to develop and implement responsible and sustainable AI practices.
We must think holistically, collaborate closely, share openly and move rapidly to ensure that AI contributes positively to the long-term prosperity and well-being of our global community.
Don't miss any update on this topic
Create a free account and access your personalized content collection with our latest publications and analyses.
License and Republishing
World Economic Forum articles may be republished in accordance with the Creative Commons Attribution-NonCommercial-NoDerivatives 4.0 International Public License, and in accordance with our Terms of Use.
The views expressed in this article are those of the author alone and not the World Economic Forum.
Forum Stories newsletter
Bringing you weekly curated insights and analysis on the global issues that matter.
More on Emerging TechnologiesSee all
Gideon Lichfield
February 11, 2025
Leila Toplic
February 6, 2025
Cathy Li
February 6, 2025
Odin Mühlenbein and Daniela Matielo
February 6, 2025
Charlotte Edmond
February 5, 2025
Nada AlSaeed
February 4, 2025